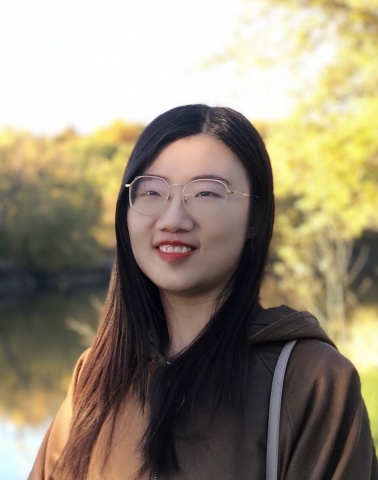
Dr. Xi Chen, working with Prof. Joe Gomes and Prof. Jun Wang, published their collaborative work in a prestigious journal, Geophysical Research Letter. Their work presents a new, machine-learning based, approach to predict light scattering properties of non-spherical dust particles. The approach will have wide applications in satellite remote sensing and climate model predictions.
https://agupubs.onlinelibrary.wiley.com/doi/10.1029/2021GL097548
Plain Language Summary
Dust particles affect both solar and terrestrial radiative transfer, but whether they cool or warm the climate is currently an open question in the literature. Accurate estimation of dust scattering and absorption properties, while critical for climate studies, is hindered by the fact that dust particles have irregular shapes and large size ranges; hence, no single method can be applied for all particle sizes and shapes. Often, a comprehensive look-up table of these properties is created by combining multiple methods. The application of such databases, however, is cumbersome and inaccurate due to the need for multi-variable interpolation. Furthermore, the look-up-table approach lacks the mathematical rigor needed to determine the sensitivity (Jacobians) of the single-scattering properties to the dust size, shape, and refractive index that are needed in remote sensing algorithms. The aforementioned challenges are tackled here by developing a novel approach within the neural network (NN) framework. This NN-based approach is fast, accurate, and able to predict Jacobians with analytical formulas. The NN model can be readily applied to the dust retrieval algorithm and radiative forcing modeling. The concept of deriving Jacobians from the NN model in this study can also be generalized for application to other problems involving gradient calculations.